Reducing flood risk for London Underground
Reducing flood risk for London Underground 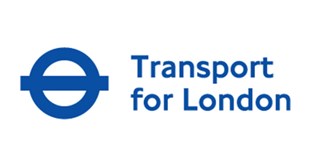
London Underground serves almost five million journeys per day. It has 270 stations, 250 miles of track and, as you’d expect, lots and lots of location data.
When Transport for London (TfL) instigated a review of flood risk across the Underground network, we worked with its GIS team to make it happen.
Previous reports had been commissioned for parts of the network, but they were in different formats and not comparable. They also each represented a single point in time.
This time, as Senior GIS Specialist Naomi Ang told us, “One of our main aims was to enable active flood risk management. We didn’t want a report that would be immediately obsolete and just filed away. We wanted to continually update our datasets and refine the analysis so that it’s always up to date and reflecting any environmental (or asset) changes.”
Modelling flood risk requires data from many different agencies – a classic case of data transformation and integration. For TfL, the challenge was increased by two factors:
- Volume of data – flood modelling requires many large datasets from many different agencies
- Different coordinate systems – while many agencies use the British National Grid to define location, TfL requires engineering-level accuracy and uses the London Survey Grid
We worked with TfL to upgrade, support and provide consultancy on their installation of Safe Software’s FME. As a result, FME is now a core tool for TfL’s GIS team.
Developing automated workflows on the upgraded FME transformed the flood risk project, as Naomi explained:
“LSG transformation was the major obstacle in the first year of the project. The new FME process has removed that and allowed me to focus on more valuable aspects of the data and analysis. We’ve found we can do much more with the data than we realised, because we have the time to focus on analysis.”
Among the benefits the TfL team has seen are:
- A 13 week per year reduction in data processing time, and
- The two-week data translation process reduced to just 18 hours.
You can read the full story – including further savings, better decision support and Naomi’s prototype predictive model – in our new case study, here.