PAI White Paper
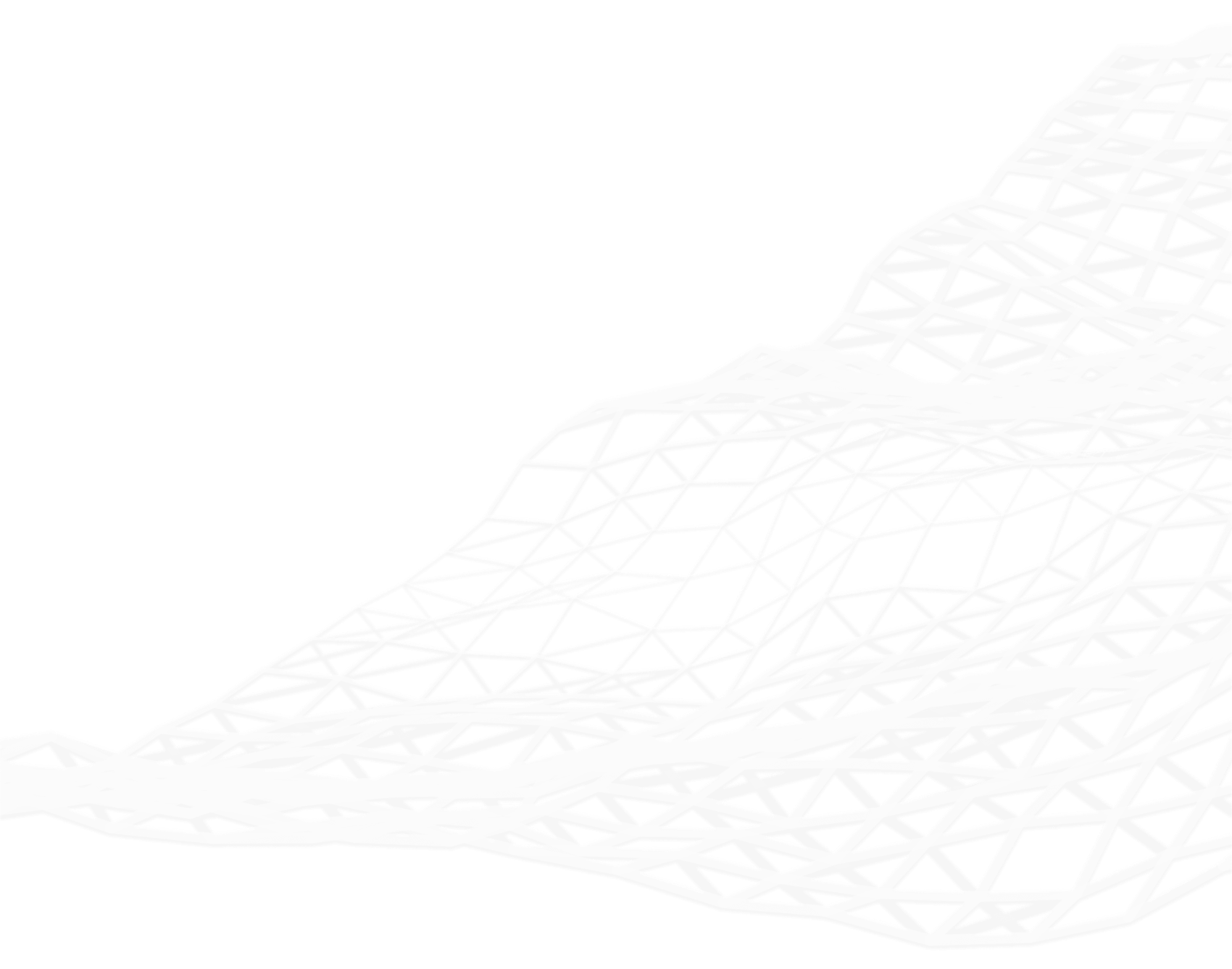
Positional Accuracy Improvement (PAI) White Paper
Mapping, as a means of communicating information about things in the real world, has been around for thousands of years. Its value has always been determined by how effectively and unambiguously it achieved this communication.
In a world where differential GPS provides centimetre-level accuracy and every smartphone includes a GPS receiver, there is an expectation that reference maps and associated data are maintained at a much higher level of positional accuracy to be fit for purposes.
As spatial datasets from various sources are integrated more frequently, it becomes essential that the positional accuracy of the data be fully understood, and improved, to avoid misinterpretation. Many reference maps were originally captured on paper at different scales. When these were digitised to create the first digital datasets, they effectively locked-in a positional accuracy deficit.
The result is that many reference base maps have needed positional improvement. Some have already been done, others are in the process. The far-reaching consequence is that any other datasets that were captured with reference to the original reference map now need to be updated to maintain their spatial relationship with the improved reference map. Examples include databases of asset locations (such as sewers, utility cables etc.) and records of land boundaries.
When a geospatial dataset is derived from a reference (base mapping) dataset – either by copying the geometries or digitising against the reference – a topological relationship, such as shared boundaries or connected endpoints, is created between the user’s dataset and the reference dataset. If the reference dataset is then upgraded with improved geometry positions, the user’s data needs to be re-synchronised with this improved reference to maintain the topological relationship. This is the process of Positional Accuracy Improvement or PAI.