Using Data to Predict and Prevent Leakage
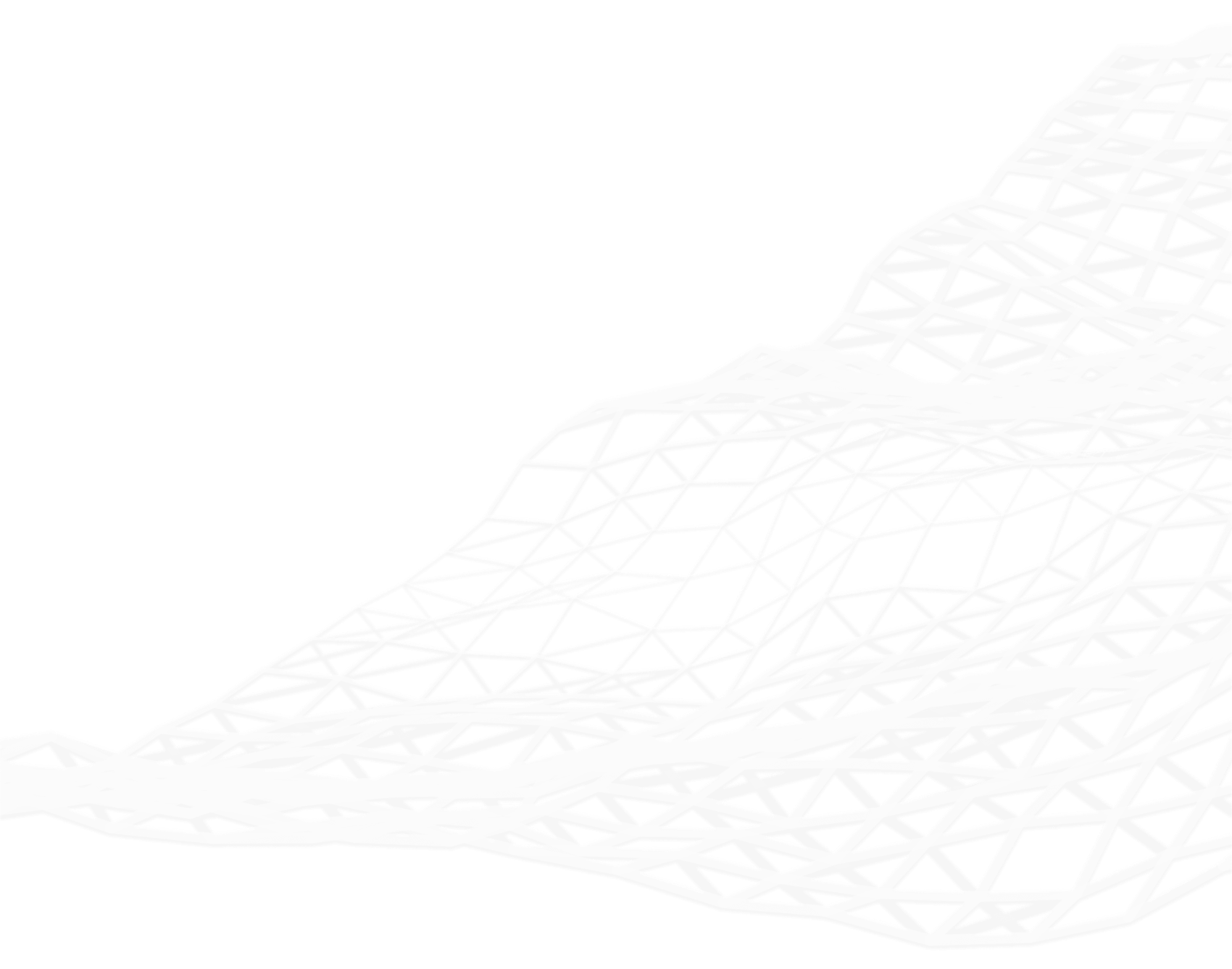
A drought has been declared across large parts of England. Temperatures have soared this year with highs hitting over 40 degrees in July. It has also been the driest July since the Met Office started recording them almost 200 years ago. Hosepipe bans have been introduced in Kent, Sussex, Pembrokeshire, Cornwall, Devon, Yorkshire, Hampshire, the Isle of Wight and the Isle of Man. Yet the public and press are asking why are hosepipe bans being introduced when three billion litres of water are being lost through leakage every day. It’s a fair question but the problem goes deeper than just fixing the leaks. It is estimated that there are 216,000 miles of water pipes in the UK. Made out of various materials, at differing elevations of pipe installation and a vast amount of ages; some dating back to Victorian England. It’s unfeasible to change all 216,000 miles of pipes so how does a water company know where to start? That’s where the 1Spatial Leakage solution comes in. It uses machine learning to accurately predict where potential leakages might occur. Allowing water companies to focus on prevention, as well supporting fixing known leaks, by predicting the exact location of the leak.
What is Machine Learning?
Artificial Intelligence (AI) techniques have been discussed for decades and Machine Learning (ML) is one part of this wide spectrum. Machine Learning is based on techniques as neural networks where the system learns from, or detects patterns from, examples and sample data. In practice, this is usually implemented alongside other approaches where you have specifically defined logic and rules. For example, with self-driving cars you could use Machine Learning to help inform image recognition (improving recognition of paths and hazards), however you wouldn’t use Machine Learning to teach driving regulations – you would dictate these in advance.
Applying AI to gain geospatial insight
Geospatial analytics are vital for infrastructure management across various modalities of cities’ assets. For example, efficient water resource management is of paramount priority to water utility companies around the world. While leakages in a water supply network amount to the loss of water as a precious resource, the leakages in water distribution systems can also lead to health and safety issues. The ability to identify high risk locations within the network, which are susceptible to a variety of damages, not only helps avoid interruptions to water supply but also allows agencies to plan for handling emergency events in an efficient way. Any disruption in the water supply network leads to an equal magnitude of disruption in other facilities of a city or town’s infrastructure such as the damage caused to roads, power supply lines, drainages, and traffic. Being able to predict any such possible disruption to water supply lines in advance, allows agencies to coordinate well while repair works are undertaken.
How we are predicting likely leakage
The key is to be able to develop a system that learns and understands the unobservable and hidden relations between various structural properties of the network, such as the material of the pipeline, along with the other parameters that render a pipe vulnerable to leakage. Such additional parameters include age of the pipe, previous history of leakage (either for a similar material of the pipe or at a given location), the length, diameter, or elevation of the pipe installation.
Our solution allows you to quickly identify the location of assets that are most at risk of leakage by recognising patterns using the spectrum of artificial intelligence, not otherwise identified by traditional methods and tools, and to make sense of the proliferation of data, making it much easier to integrate more data sets for use in analytical assessments.
But what is key here is the data, which sits at the heart of any organisation.
How to train Machine Learning models
A lot of data is required to train ML models, and anyone doing this should ensure that this data is clean, correct and unbiased so we don’t teach the model the wrong thing.
We use our rules engine to create our model, but we also use the same rules engine on your data to verify, clean up, merge and combine it with different sources and ensure that it is consistent, compliant and complete.
Rules based systems can be used to encode this geospatial information into numbers used by the ML processes such as size, shape, proximity or other geospatial interactions with the surrounding data.
Neuro symbolic AI acknowledge that rules and machine learning combined are more powerful as it allows the guiding logic of a person explicitly defining rules alongside the fuzzy inference benefits of machine learning from lots of data.
Collating the data in a meaningful way from which to draw predictions
Machine learning is closely coupled to 1Integrate to provide a complementary capability. Together, the ML and rules-based capabilities enable both scalability and generalisation capabilities that any application can leverage. By using the 1Spatial Leakage solution, it becomes possible to perform geospatial predictive analytics based on machine learning. This allows us to consider other supporting data such as the type of soil, or proportion of intersections with roads and railways which make pipe breakages highly likely.
While 1Integrate, as an efficient rule-based engine, is capable of geospatial analytics, the trained machine learning model uses the data and identifies correlations and patterns across various attributes of the pipeline by means of probabilistic learning and deep learning neural networks. As a result, 1Spatial’s Leakage solution can be used in a dynamic manner by water utility companies to chart out a leakage probability map and preventive maintenance plans. Tests against past data show very promising results using this approach of ‘Predictive Geoanalytics’, where it is effectively used to address and solve the problem of predicting possible leakages in water pipelines in advance.
Our solution has been designed with subject matter experts and combines a rules-based approach with machine learning.
The solution is cloud-based and has a very simple self-service interface, to easily upload data, track and be notified of its progress as it is being processed, and then download the results. We tell the rules engine how the data should be, this validates the data before calling our machine learning process.
Our machine learning process scores all the individual pipes that you uploaded, regardless of whether they are within a District Metered Areas or network zone. The results which you download help to locate pipes of high leakage probability saving time and resources by directing repair engineers to the right location.
So where does our leakage solution fit in?
Two main use cases
We know that finding a leak can take a while and, by using the leakage solution, our goal is to reduce that by giving each pipe a score which enables engineers to visit pipes with a high-risk score first.
The same output can be used to help prioritise the strategic asset management of where to upgrade pipes at high probability of leakage first.
Watch our On-Demand Webinar
In this webinar we discuss how data can be used to predict and prevent water leakage with Jeremy Heath, Innovation Manager at SES Water and UKWIR’s Leakage Programme Lead.
Watch now