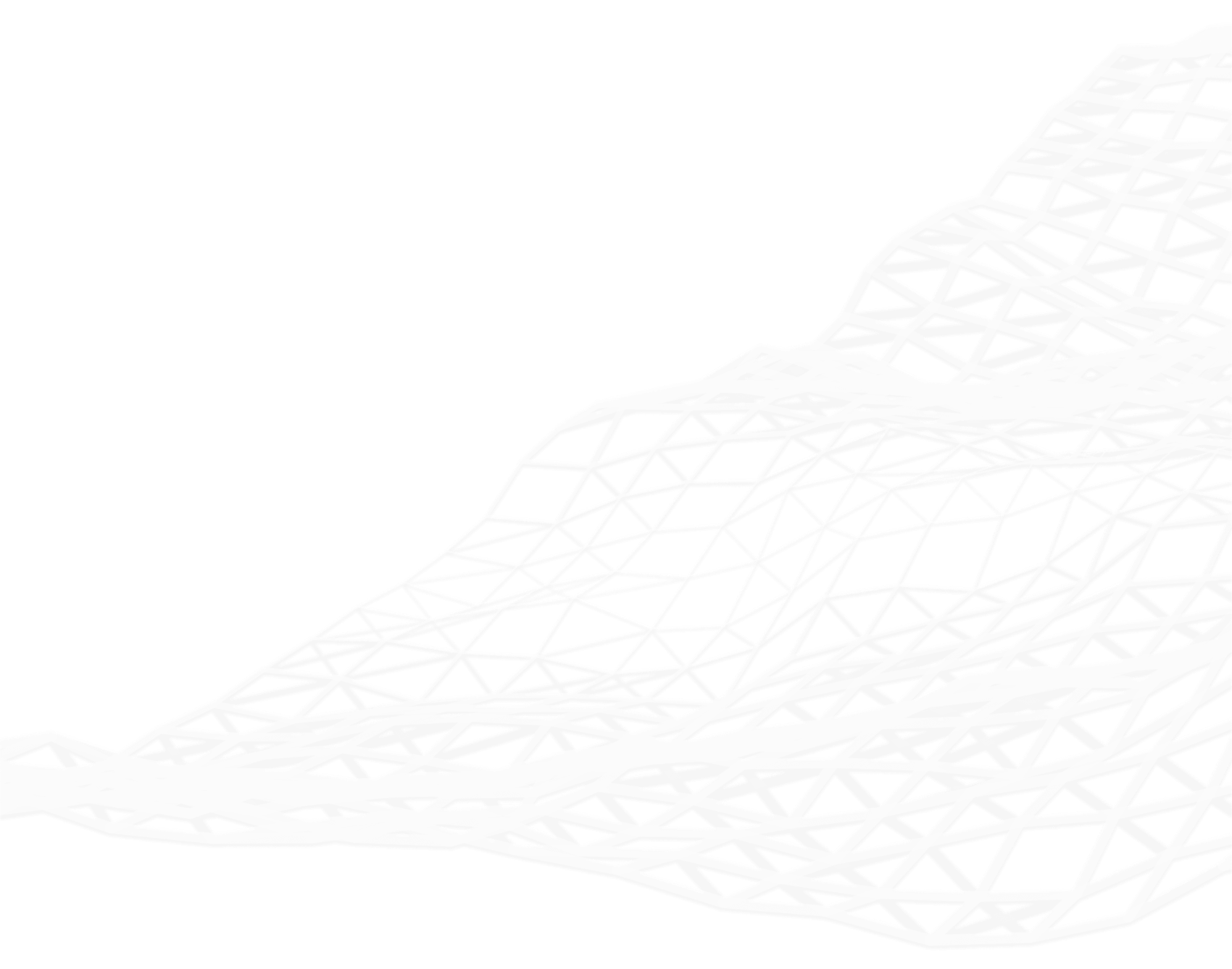
Data Quality
Make your spatial data fit for purpose
Making your geospatial data fit for its intended use is central to effective data stewardship.
To achieve this you first need to be clear about what “fit for purpose” means in your specific context.
Our approach is designed to help you discover and precisely define your data quality requirements, to check how your data conforms to those requirements, and then to cost-effectively bring your data up to standard.
Speak to a data quality expert
1Spatial has been at the forefront of data quality and governance for the past 30 years. We've helped more than 1,000 customers develop strong data foundations, unlocking the value of their data and enabling them to make critical decisions.
Speak to a data expertWhat is data quality?
Data quality means ensuring your data is fit for its intended use.
No data is a perfect reflection of the real world, so organisations typically decide what level of quality is acceptable. This could mean defining which rules are mandatory or optional and the acceptable conformance levels for each rule.
The key is understanding what you need.
Working with clients, we often help them to determine exact business requirements to allow them to define quantitative quality metrics.
How do you measure spatial data quality?
There are two main elements to be considered in determining the utility value of the data. The first is the direct impact when data quality is below expectation, and the second is the lost opportunity cost which, although harder to measure, can often be the more significant.
Although it may be difficult to quantify the monetary value of spatial data quality, we can readily see the impact it has in our daily lives, for example:
- Road network data must be properly connected for satellite navigation systems to function correctly.
- Cadastral information must be accurate to support a functioning property market, provide security to allow investment, facilitate provision of services and enable valid taxation.
- Built environment information must be correct in order to support urban planning, environmental protection etc
- Utilities infrastructure information must be accurate in order to ensure safe and effective asset management and maintenance.
Spatial data is particularly sensitive to two specific quality measures:
Accuracy
- Positional – the geometric representation of the location and shape of the feature
- Topological – the spatial relationships between the features properly reflecting what exists in the real world (e.g. are the pipes connected?)
- Temporal – how a feature changes over time (e.g. a house extension or coastal erosion)
- Thematic – the classification (e.g. is it a river or a canal?)
Completeness
- Missing – is required data missing e.g. does a pipe have an appropriate connection.
- Detail – is the required level of feature detail captured e.g. is the land parcel representation suitable for use in a property transfer of part, is the building represented in CityGML LOD3.
The richness and complexity of spatial data models representing real-world features demands that the quality measures ensuring fitness-for-purpose are comprehensively determined and that robust, trusted processes to verify compliance are put in place. This allows a transition from fearing that the data is not of high enough quality – and suffering the effects – to knowing the exact level of quality and being able to measure and improve it.
Free e-Book: The Little Book on Spatial Data Quality
- The cost of poor data and how to ensure a return on your digital investment
- How to realise the spatial data opportunity
- Important considerations for managing data quality
- The process to follow for data improvement
- 6 data excellence principles
Download now
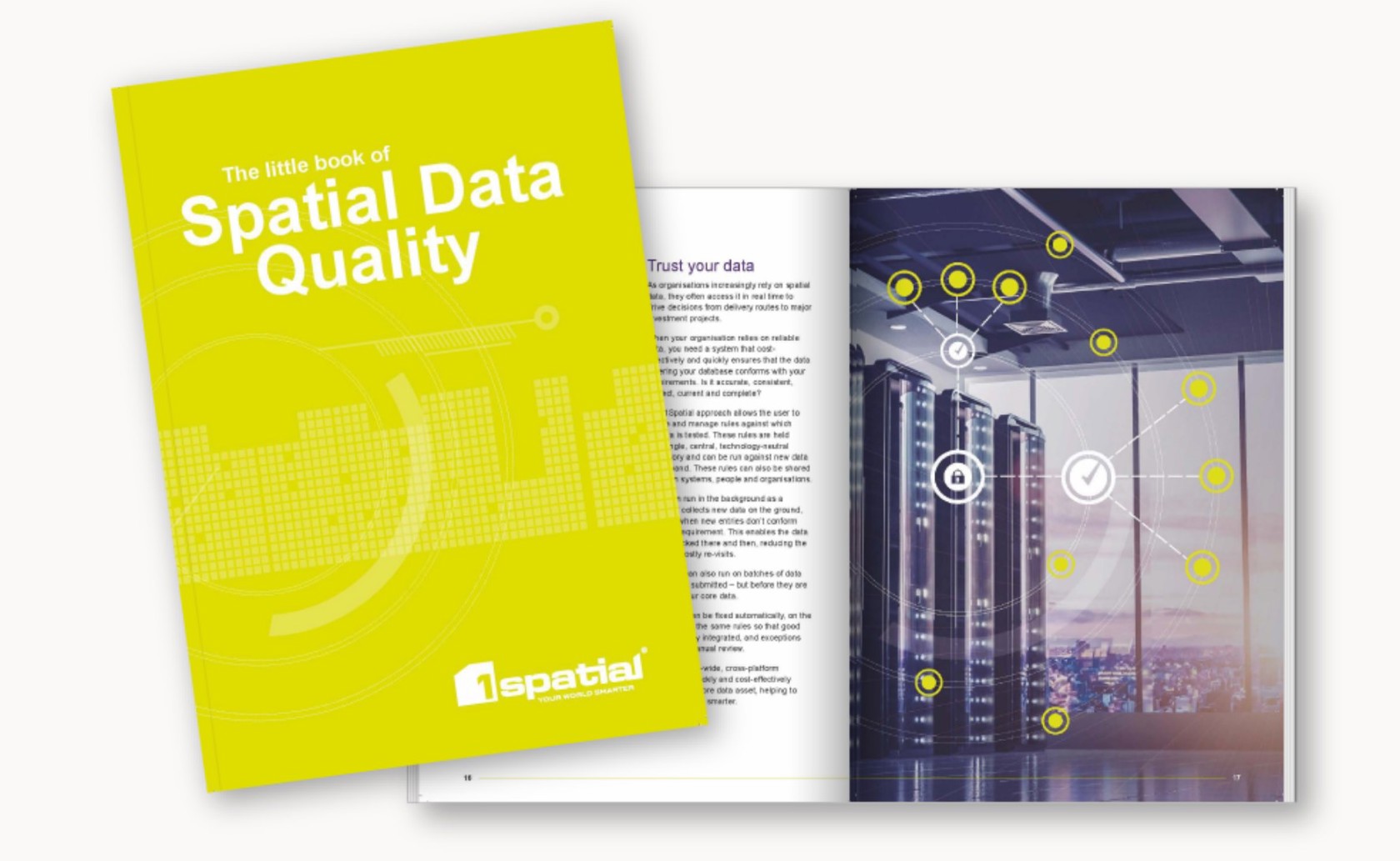
How 1Spatial can help
1Spatial’s core business is in making geospatially referenced data current, accessible, easily shared and trusted. We have over 30 years of experience as a global expert; uniquely focused on the modelling, processing, transformation, management, interoperability, and maintenance of spatial data – all with an emphasis on data integrity, accuracy and on-going quality assurance.
We have provided spatial data management and production solutions to a wide range of international mapping and cadastral agencies, government, utilities and defence organisations across the world. This gives us unique experience in working with a plethora of data (features, formats, structure, complexity, lifecycle, etc.) within an extensive range of enterprise-level system architectures.
The 1Spatial approach
We can work with you to suit your operational needs and requirements. We can provide our solutions and expertise as a service approach or alternatively you can use our tools yourselves. Either way we start with a detailed Data Quality Assessment. This includes a data discovery process that defines precisely your data requirements.
The discovery process is followed by Data Conformance Checking that assesses your existing data against your requirements to provide a detailed, baseline analysis of your data and any gaps.
The Data Quality Assessment can then be repeated in an ongoing basis as a persistent Data Quality Management process in which our automated, rules-based tools can cleanse your data, maintaining it continuously up to standard.
Performing a Data Quality Assessment and addressing any issues raised, are traditionally labour-intensive, time-consuming manual processes.
Read the Blog: How to Deliver Continuous Data Quality Improvement
Find out how to automatically and continuously validate, correct, transform and integrate your data at scale with our patented rules engine.
Automated, rules-based data validation
The 1Integrate rules engine solves the issue of managing the quality of data in one or more databases. Ensuring good data quality, which is also referred to as Master Data Management, is an issue for most organisations, especially where databases are large, complex and interconnected with other systems. Bad data quality reduces the operational efficiency of organisations and prevents effective decision making.
The 1Integrate Rules Engine solves this issue using a rules-based validation process which checks and cleans the data in order to measure, improve and protect the quality of the data and hence improve the operations, decisions and software implementations that depend on it.
Benefits of automating your data quality process
- Cost-effective and efficient – Data rules apply the best judgement of your experts, consistently, automatically and on-demand. Our solution can quickly find and fix the majority of data issues, identifying any remaining complex issues for the attention of your experts.
- Collaborative – We work with the users and creators of your data to agree your real requirements. Our user-defined and user-managed rules make data quality an explicit process that can be discussed and adjusted with business needs.
- Consistent – Once defined, data rules can be run at any time, across your organisation to produce a consistent measurement of quality and to apply repair and cleansing rules in a consistent, repeatable way.
- Thorough – We assess accuracy, consistency, correctness, currency and completeness of your data against requirements. And, our rules ensure the same, thorough assessment can be repeated as required at any time, on any data-set, anywhere in your organisation.
- Enterprise-wide and technology-neutral – Using a single, central repository of user-managed rules means you can run the same rules across all of your data sets, regardless of technology platform or format. It also means avoiding the risk of being locked into ageing technology by expensive bespoke programming.
No 1 AIDU feeds forces with accurate aeronautical information
“1Spatial has a good understanding of what’s required and over the last couple of years we’ve developed a very collaborative relationship. It’s helped us massively. Not only does it save us a lot of time and money, but it means we end up with the best solution for our needs.”
No 1 Aeronautical Information Documents Unit