What lies ahead for 2024? It’s all about Geospatial AI
Navigating the Future of Automation, Drones, and Data Aggregation
I predict that all industry predictions will focus on AI (Artificial Intelligence) and it's hard not to with so much new hype last year. In fact, in my previous years’ predictions, I highlighted that we would see some use-cases for AI in the industry grow while others fall short, depending on the available data. What it didn't predict was the explosion of interest in Large Language Models made accessibly by OpenAI's ChatGPT and this will certainly help boost many tasks involving humans interfacing with machines - but it is still a 'language model' and not a spatial model. This means that it can help empower users for tasks such as documentation, code, and script writing, or helping interact with complex systems such as for analytics or schema matching, which are generic tasks and not unique to the Geospatial industry.
Meanwhile, truly geospatial uses of AI will be in two principal areas:
1. Digitising unstructured data such as imagery, point clouds or PDFs into structured spatial content
This has been happening steadily for a long time though it has never quite achieved the levels of accuracy and automation that were hoped for. It's used more for anomaly detection (e.g. does the video show a crack in this pipe? Do these trees overhang the railway?) but maybe that continued improvements will make mostly automated data capture and (more importantly) data update and maintenance more achievable.
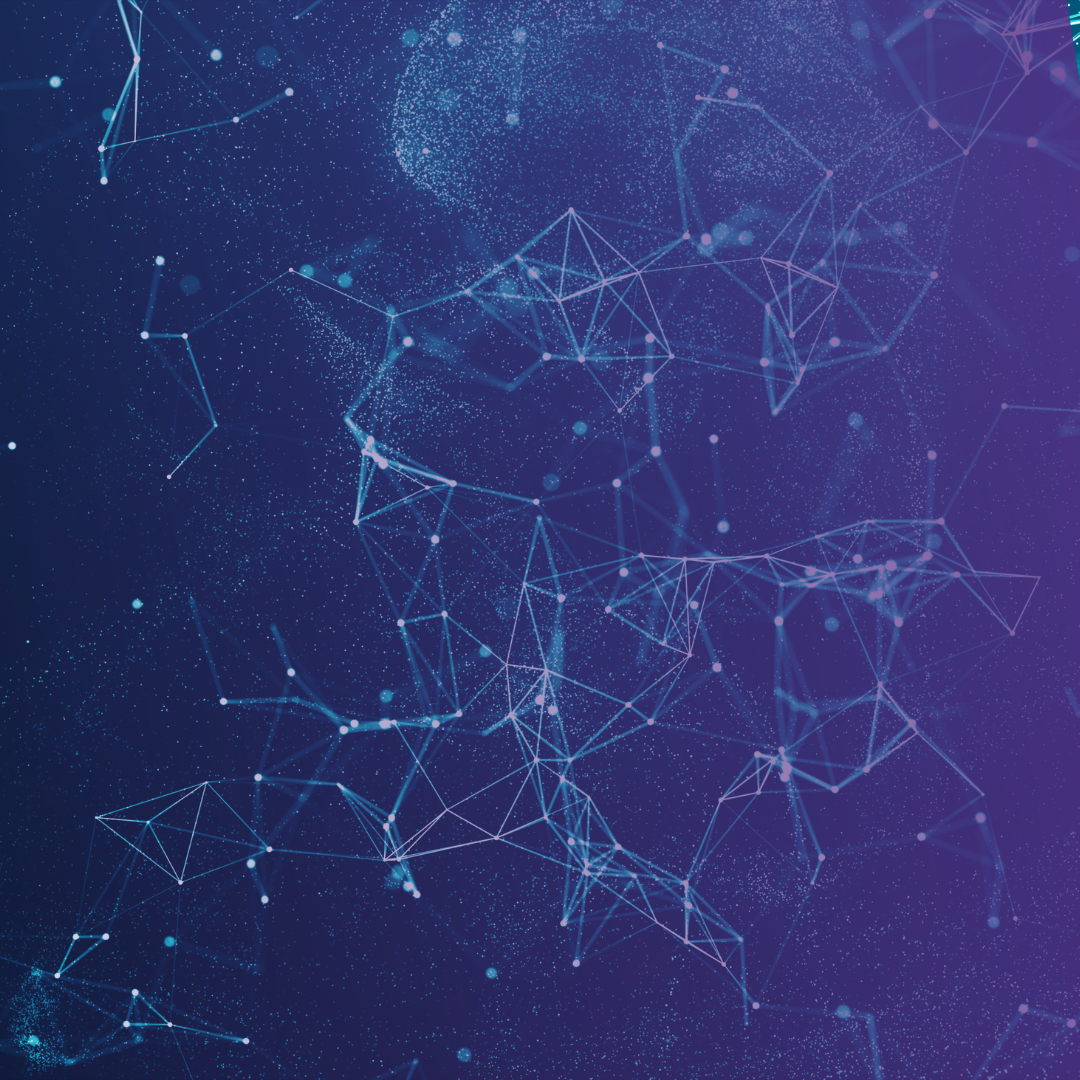
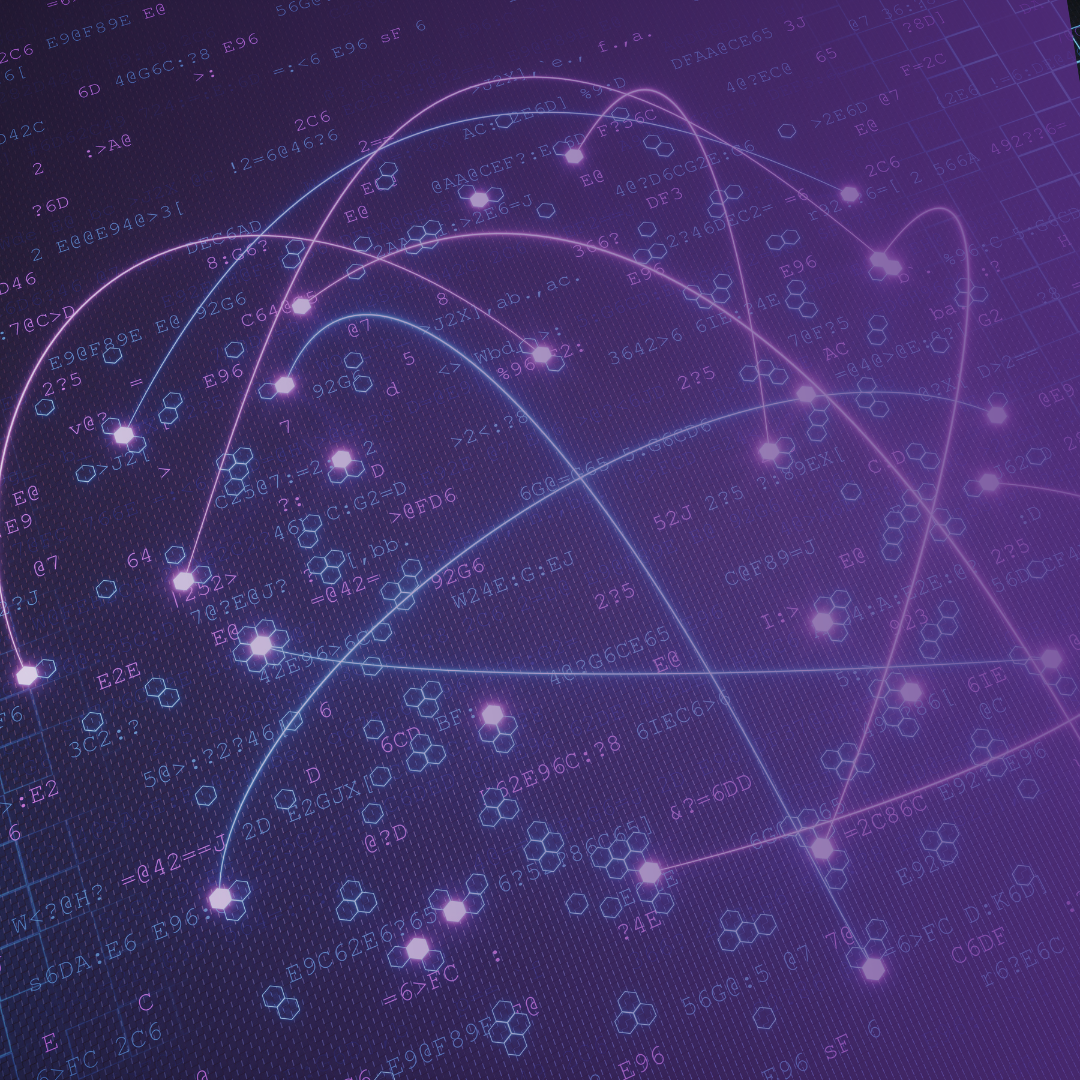
2. Using structured spatial data for analytics and inference
This is an area of opportunity to automate more tasks that are currently manual and require good-quality structured spatial data as input, as well as many examples of 'the right thing' to train the models. We expect to do more of these types of projects this year and maybe one day, a tech giant will create a global 'Large Spatial Model' equivalent to a Large Language Model, to represent the global natural and built environment - which would make these projects even easier.
Drones Revolutionising Data Capture: A Catalyst for Structural Data Enhancement
One method of capturing this unstructured or semi-structured data is using drones which for several years have been the highlights of geospatial hardware shows. They are widely used for human inspection via cameras, or for point cloud capture for projects but mostly just for human visualisation. If the AI techniques described above improved automated management of structured spatial data, then this would drive the use of drones for not only human interpretation, but structured data capture so one improvement would unlock the other.
In the meantime, there is still a disconnect between the data produced by the design and build phases of construction - held in CAD formats, drawings or point clouds - and the data needed by large scale data management systems of record. The handover and adoption of this information is a big driver for projects that we have been involved in over the last few years. We are seeing a tipping point where the automatic validation and integration of this data is now the norm, so more organisations will adopt this approach. Some projects such as the National Underground Asset register are no longer worrying about 'how do we ingest, integrate, maintain and share this data?' but 'what are the future use-cases for this hugely valuable and up-to-date structured data asset?'.
Ensuring Data Quality in the Era of Automation
The growth of automation in data capture and data ingest projects also drive the need for measuring and protecting data quality to ensure that automation does not lead to loss of data quality, which might otherwise have been spotted by the people capturing the data. Automation of data quality alongside automation of data capture means the data is then suitable for powerful use cases such as underpinning digital twins and smart cities. These large-scale data aggregation projects mean that there will be a better data framework from which these smart uses can flourish, and we hope to see more of that in the coming year.
Data aggregation hubs might only be a steppingstone towards a federated data mesh approach. Aggregating data that is mastered in many different systems by physically mirroring it in an up-to-date data hub is great to get consistent data in a consistent structure which provides a single system to manage resilience, performance, security, and role-based access. But there will always be a lag between what is stored in the hub vs the latest version of the data which might be updated on an hourly basis. A federated model in which the data is pulled live from each data mastering organisation's system would provide an even more up-to-date version of the data.
Navigating Challenges: Metadata Catalogues and Data Federation
In the shorter term this is usually achieved using metadata catalogues which can be searched to find and link to relevant data which can be streamed or downloaded. This catalogue approach allows the data to remain in the mastering systems, but they are not usually made available in a consistent structure or format, so it is harder to aggregate this data for use.
Data federation is harder, especially when an agreed structure is needed for virtual aggregation, because it requires an agreement on the structure and encoding of the data as well as a high level of technical maturity at the data custodian to provide live services which are scalable and secure. While there are good standards for data sharing from organisations such as the OGC (Open Geospatial Consortium), and good examples of live data feeds being used in production, it will be interesting to see whether more widespread secure data federation is progressed this year - possibly not yet.
All these capabilities are underpinned by web connectivity and therefore also at risk of hacking and disruption. The AI techniques described above which can automate positive outcomes, can also be used to speed up and empower cyber criminals, terrorists and 'state actors' for negative outcomes and so the ongoing security arms race will continue at full speed with continual upgrades, testing and best practices. Whether there are any seismic changes in the security area we don't know, but it will be an ongoing discipline that needs to be kept up with to sustain and improve confidence in the systems to ensure that they can continue to be connected in a trusted and secure way.
Looking Ahead: Automation, Efficiency, and New Opportunities
In summary then, many of these developments enable more automation, and automation drives efficiency and opens up new opportunities so we should see various outcomes becoming real this year: Automated AI Data capture experiments will start to show whether they are viable, New data aggregation projects will start to automate ingestion by enforcing rigorous data checks and existing aggregation projects will start to benefit from leveraging their data in new and innovative ways.
Author: Seb Lessware, Chief Technology Officer, 1Spatial