3 steps to optimising your Data Supply Chain
In the age of data-driven decision-making and digital consumption, data is arguably a business’s most valuable asset.
We’ve previously compared data to oil, but now we like to say that “data is the new honey” – as to really derive value, it first needs to be collected and processed by multiple people, and often many technologies.
So, it is vital that we collaborate across the organisation and its supply chain for data, in the same way we would any other supply chain – transforming raw materials (either physical or digital) to finished products or intelligence. Likewise, data supply chains should be optimised for quality, efficient processing, security, and to deliver the maximum value to consumers.
Common challenges in the data supply chain
1. Different data goals
Let’s start from the beginning – when harvesting raw data in the field. Data is typically collected with a specific use in mind, and even for a specific type of user or persona. For example a surveyor might digitise a new housing estate with the goal of recording building footprints. They might not be considering building access points, address data or other metadata relating to the use of the buildings. Perhaps it is a multiple occupancy building? Or it might be a commercial building such as a corner shop. These details may not be accurately recorded as they are out of scope of the data collection programme, but they might present huge value to other data use cases (for instance for the purposes of tax collection in multiple occupancy buildings).
2. Varying techniques and formats
When data is being collected by different parties, there will often be subtle differences in format or schema. This could be down to tooling, capture techniques, varying levels of quality control with each supplier organisation, or simply the habits of a particular data collector.
Conforming to given requirements is crucial at the data capture stage. There are often standards and specifications that data must adhere to, ideally during capture and before data submission, but it is not un-common for the data to be checked after creation, and upon receipt. The implications and risks of not conforming to these standards at all are delays, re-surveys, inefficiencies, and ultimately - increased costs.
3. Volumes of data
But the challenges don’t stop at this end of the supply chain – once collected, the data needs to be processed. The volumes of data being collected grow exponentially every day, and this can be a monumental task for data aggregators and processors to address.
Think of organisations such as the US Census Bureau, who every 10 years must collect data from all US citizens, combining boundaries and road data from state, local and tribal governments alongside addressing data from the postal service. Similarly, the UK’s National Underground Asset project (NUAR) has around 650 asset owners (users), who each might have around a dozen groups of data. Some of those groups run from 100s to 100,000s of features.
4. Data in flux
Besides collecting new data, another challenge is changes to existing assets, especially if a third party incorporates that data asset into its business process. This is where the feature lifecycle and unique identifiers are key. For example, if a field or pipe is split in two, the ID of the original feature may stay with the larger feature, and a new ID would be created for the smaller. What other systems or data use those identifiers? Do other systems need to be informed of the change? It is crucial to have business processes that perform appropriate matching with other business data to deliver effective master data management.
If these large quantities of data are not processed quickly enough, consumers will find the data is already out of date or “stale”, and all that hard work will have been wasted. Likewise, if the data is not stored in a structured and useful way, how can data consumers find what they need to know, make informed decisions, or even know what is or isn’t available to them?
5. Interoperability
Interoperability, or the ability to exchange information securely between systems, is crucial to the access and delivery of your data throughout the supply chain. This can be extra challenging to ensure across multiple systems, especially where legacy technology may be involved. Any process you design for your supply chain must be adaptable and scalable for ever increasing data loads and security requirements.
US Census Bureau
Read how the US Census Bureau saved $5 billion due to automated data integration across the supply chain.
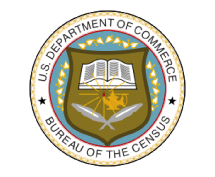
The advantages of a strong data supply chain
Beyond simply avoiding the problems we’ve outlined, building a strong supply chain for data also offers new opportunities, especially if we’re considering data as a product in its own right (which will deliver continual value for the organisation).
1. Re-use and reduce
Maximising data sharing and re-use allows for a greater variety of end-products, accelerating innovation and may help you to address new markets. It can also reduce the amount of work having to be carried out in the field – no need to unknowingly collect data twice if your processes and pipeline are transparent enough. Ensuring your data is correct and complete at the time of collection means fewer site visits, and saves time, money and frustration!
2. Integrate and innovate
Location can often be the key to tying together different data sets, as everything happens somewhere.
Improving your data discoverability with good structure and indexing can be the key to innovation. After all, you need to know what ingredients you’ve got before you can come up with new recipes. What if the key seasoning ingredient was hidden at the back of your cupboard and you never knew it was there?
The collation of all assets in one format and hierarchy, enables you to identify real-world assets in a structured way that all stakeholders can locate and understand.
3. True and trusted
Establishing a dependable data foundation is a mark of success. Enforcing data quality standards can help you easily demonstrate compliance when required, and establish that your data and products can be trusted. The closer your data is to “live”, the more valuable the intelligence that can be leveraged from it.
It is also important that you don’t default to accepting the bare minimum just to get asset data incorporated into your data management platform. Strong processes, such as automated rules, mean you can enforce that the data submitted to you is of a sufficient standard.
How 1Spatial helped the U.S. State of Minnesota with their NG9-1-1 Transition (10-Minute Watch)
“We could not have accomplished this without the support of 1Spatial.”
9-1-1 Program Manager, U.S. State of Minnesota3 Steps to optimise your data supply chain
So how do you go about creating that ideal data supply chain? Here are 3 of our tried-and-tested keys to success:
1. Data assurance at all stages of the data lifecycle
Quality control and data assurance shouldn’t just be left to one stage of your data supply chain – it should be embedded throughout.
- Data collection
Validation at point of collection (“right first time”) is of course helpful at minimising wasted effort and ensuring you’re capturing everything you need. By using mobile field collection tools such as 1Edit or 1Capture, you can also simplify the data capture process and enforce automated attribution.
- Data submission
There are other ways you can assure quality at the point of data submission. 1Data Gateway is a simple web portal, built on top of a powerful rules engine, where data submitters can check that their data complies with configurable business rules as determined by the data controllers. Non-conformances can be flagged instantly for correction. The tool can also perform automatic corrections based on predetermined settings. Combined with ‘change only’ updates, 1Data Gateway eliminates the need for constant manual fixes to the data. Accessible via a web browser, it is intuitive and easy to use for the entire supply chain.
Read more: 1Data Gateway - The Self-Service Web Portal for Data Submission, Validation and Correction
- Data organisation and integration
Further down the chain, at the point of data organisation and integration, organisations should enforce business rules to ensure ongoing data quality, consistency and validity.
Data should be centralised from many different formats and locations and transformed to conform to a central schema. Once centralised, automated rules and actions can be performed across the entire collection of assets with automated rules engine such as 1Spatial’s 1Integrate. These rules can also be centralised with 1Integrate.
In some cases, it may even be useful to designate special sets of quality rules for specific data production.
2. Leverage analytics
Business Intelligence (BI) and analysis tools such as dashboards are invaluable for identifying weaknesses and opportunities for improvement in your data supply chain.
Metadata can be used to identify data capture methods or suppliers which may be underperforming compared to others, or to highlight business rules that are being failed the most often. Only once you’ve identified these problems or opportunities can you take action to address them.
Patterns can also be identified in the data itself, perhaps leading to new business rules you want to enforce across all datasets.
3. Automate validation, transformation and correction
Automation can be introduced in more places than you might expect. Breaking down complex manual processes into repeatable business rules is 1Spatial’s proven expertise – we’ve even managed to codify the “red book” of traffic management rules, automatically producing traffic plans in minutes that would have taken hours or even days to determine by hand.
Reducing processing time is key to keeping your data up to date, and accurate. Keeping your data compliant to a standard, model or schema ensures data is consistent and interoperable across the whole supply chain.
Freeing up people from manual work should not just be considered a time and cost saving exercise. It is an opportunity to undertake more difficult or innovative work that can’t be automated, make the most of human expertise, and to strive for continual improvement in your processes and data products.
Watch now: The Road to Smarter Asset Information Assurance
Learn how to improve your asset information journey with Nicola Pearson from the Government and Industry Interoperability Group, Emma Walker from the Environment Agency and 1Spatial's Adrian Porter. Watch the webinar on demand and learn more and hear EA's approach to asset information management.
Watch now