Spatial Data: Making Management Cost-Effective
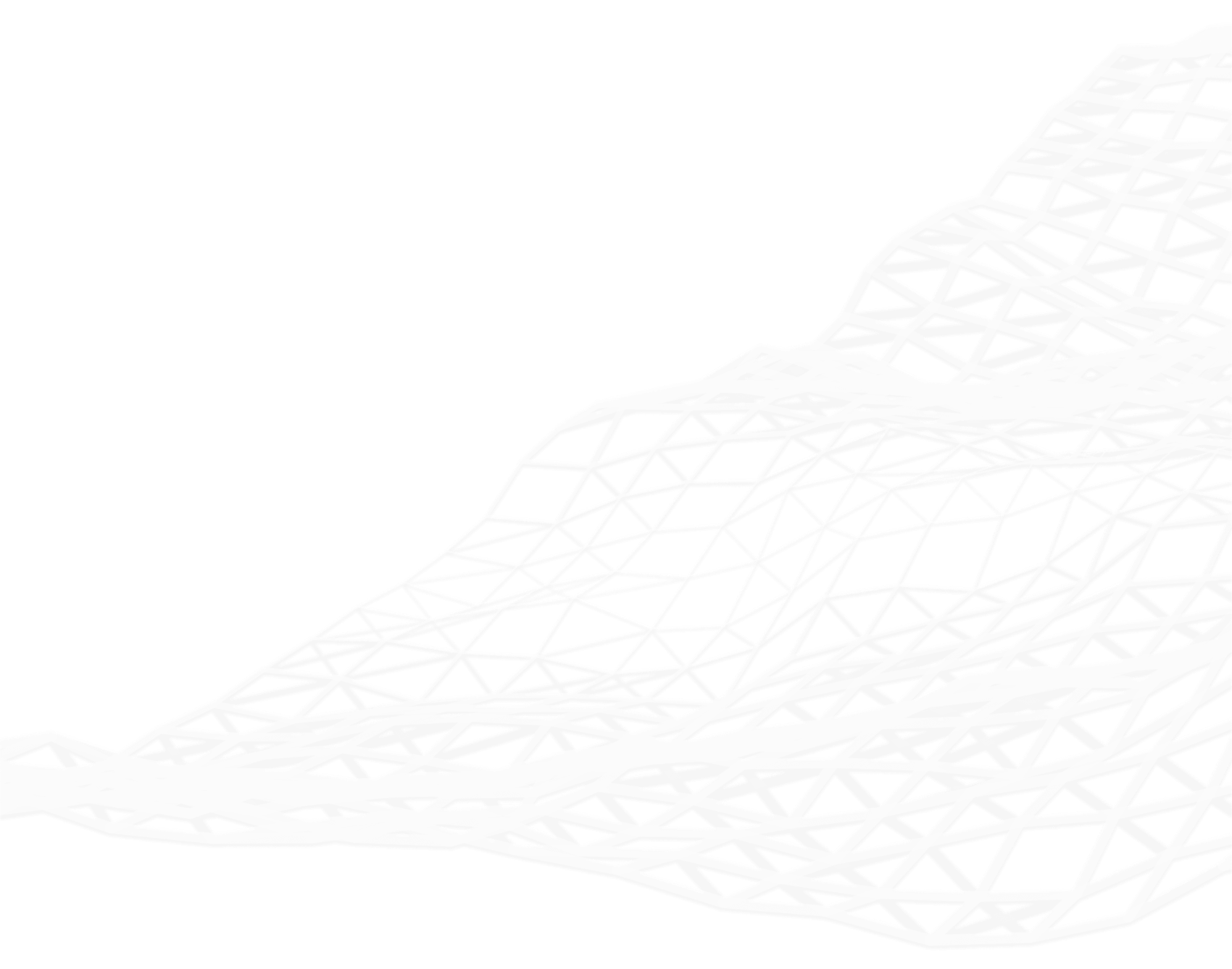
Spatial data: making management cost-effective
Last time, we looked at putting location data at the heart of business decision-making. But, how can you make your data-management efforts as cost-effective as possible?
Data management should be a process, not a project
The key to cost-effective data management is to treat it as an ongoing process, not a one-off project.
After all, spatial data is constantly changing (through data edits or bulk import of new data), as is the world it represents. Business requirements change, too. Data standards evolve, so does business strategy. Organisations upgrade their systems and enter new data-sharing partnerships.
Tasks like data cleansing just can’t be effective if they are run as discrete, often ad hoc, projects. Yet, too often, that’s exactly what organisations do. As a result, some parts of their data will be inaccurate for most of the time.
By contrast, automating data maintenance as an ongoing process means that data is always in a known state and ready to use. This simplifies stewardship and builds organisational trust in the data – critical, as firms increasingly rely on spatial data to inform real-time decisions.
At the same time, rules-based automation frees experts to focus on value-add tasks and reduces the risk of manual error.
An automated approach, with user-defined and user-managed rules, can run in the background right across the enterprise and all its data, from inception to obsolescence.
Rules can run on data at the point of collection, before acceptance into the core data set and regularly to prevent or fix accidental corruption by users.
Data quality is enforced along the way, not treated as an after-thought.
Quite simply, automated, rules-based data maintenance makes your data smarter, in a more cost-effective way. Data becomes more valuable, and the return on investment higher, when a data set is maintained for ongoing interrogation.
Data excellence principles
Too many data management initiatives run aground by targeting the most difficult problems, rather than considering where the return will be greatest.
We always recommend that a data maintenance strategy is considered in the context of six data excellence principles:
- Embrace automation
- Ensure repeatability and traceability
- Design simple solutions to difficult scenarios and avoid unnecessary technical complexity
- Target the typical, not the exceptional, to maximise value
- Adopt an evidence-based decision-making process to create business confidence in the outcome
- Collaborate to identify issues and work towards a solution
We find that a strategy built on these principles and implemented as an ongoing, automated process is the most cost-effective way of achieving the Three Cs of spatial data management: Control, Consistency and Compliance.
Next time, we’ll look at three specific data management scenarios: data migration and transformation, data enhancement and data integration.
To learn more about spatial data management and how successful businesses have succeeded with an automated, rules-based approach, download our Little Book of Spatial Data Management, here.