4 Predictions for Geospatial Data in 2023
Seb Lessware, 1Spatial's Chief Technology Officer, rounds up his view of the top trends for geospatial data in 2023
My last couple of geospatial data predictions included the natural wax and wane of machine learning projects as they succeed or fail, and we have certainly seen that happening in all industries: Eight years ago, the hype around machine learning for self-driving cars was so intense that I wondered if my kids would even have to learn to drive, and here we are, eight years later, and saving up for driving lessons.
In our industry we've definitely heard of machine learning projects stopping or reducing their scope to those specific cases where the outcomes are good enough, usually because there is sufficient input data of high enough quality. However, there are still good examples of successful geospatial machine learning projects happening.
1. Using digital twins to actually model asset performance
Digital twins will continue to be a hot topic but we will see wider acceptance that a Digital Twin is more than just a realistic-looking 3D view of something: A Digital Twin is intended to model the current performance of your asset as measured by up-to-date information from real world sensors. This allows predictions, simulations and analytics to be based on the actual current performance of the asset - which has inevitably been degraded since its construction - rather than the theoretical 'perfectly performing' asset that was originally installed.
2. Improved focus on data foundations
This appetite for digital twins has focused the need for solid data foundations to which the sensor readings can be associated and many organisations will continue to improve the quality of these foundations as the benefits of doing so become more measurable when driven by these hot-topic aspirations.
3. Federated data hubs versus data aggregation hubs
Building Information Management (BIM) is about recycling the data produced during the construction of something in order to help with the ongoing maintenance of the asset. This leads to the need for data aggregation hubs that funnel data into central enterprise asset repositories - and we have seen growing demand for this over years. Some organisations want to evolve this from a central hub of data to a federated 'data mesh' in which the data contributors maintain their master version locally and it is all just referenced centrally rather than copied centrally.
This has been happening for a long time for geospatial data via map services and feature services but often only for human consumption and less so for automated analysis, especially with asset management data. Will we see federated hubs spring up in the next year for asset data? Unlikely yet, because the aggregation approach is still happening, but eventually, federated data may become more common and when it does, it could provide an incentive for more 'linked data' and for the use of graph databases as a flexible way to manage the relationships between assets in these federated datasets.
4. Increased reliance on automation and self-service capabilities
If economic headwinds are going to put pressures on budgets then that only strengthens the need for automation and self-service capabilities, which will grow and evolve. Other more speculative innovation projects may get delayed in favour of the more short-term and tangible results, for now at least. That’s a short-sighted approach so hopefully it won’t be that way for too long If it does happen.
Unlock the value of location data for your enterprise, at scale
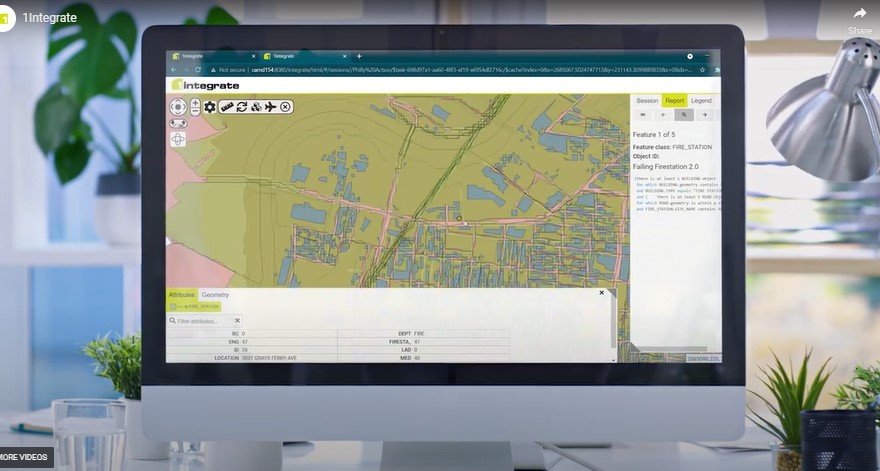
1Integrate automatically checks, corrects, controls and transforms your data.
1Integrate powers some of the largest and most complex geospatial data sets in the world, including Ordnance Survey Great Britain, and the US Census Bureau.