Why Managing Spatial Data Quality Requires A Data Governance "First" Approach
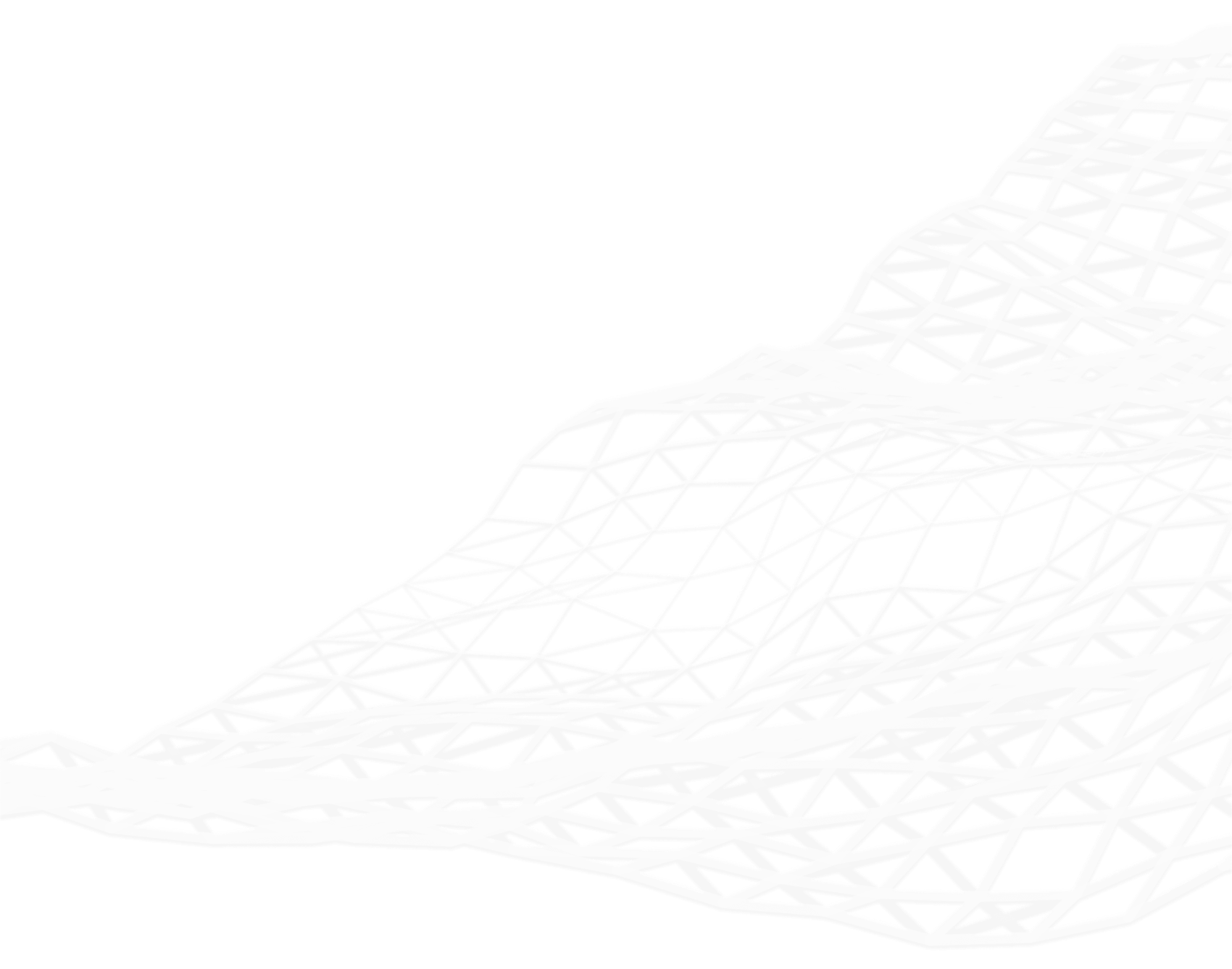
With the vast amounts of geospatial data generated every day, it’s unsurprising that there is a general lack of effective governance over these data assets.
Without data governance however, the quality of spatial data is often misunderstood and therefore mistrusted. If you are looking at using spatial data across your enterprise, it is important that you have a solid data governance plan that will help to ensure optimal spatial data quality.
How many of these questions can most organisations answer?
- How much spatial data the organisation holds;
- Where all the spatial data is being stored;
- The content and format of that spatial data;
- Who is responsible for the spatial data;
- The accuracy and completeness of the spatial data, and
- How it relates to other data sets across the organisation.
The rise of data governance
Over the past 7 years, however, there has been a meteoric rise in data governance as a discipline. In fact, interest in the term ‘data governance’ – has quadrupled since 2015 according to Google Trends.
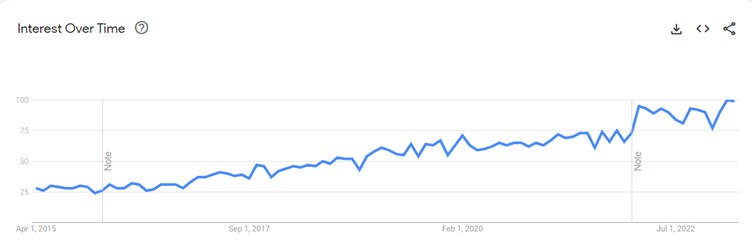
Free webinar: Achieve better data governance with 1Integrate
Join us for this practical discussion of how to achieve better data governance by improving your data management with 1Integrate.
Register nowHow do data governance, data management and data quality fit together?
First, let’s start with some basic definitions.
"Data governance” sets out policies, roles and responsibilities, standards, metrics and procedures for managing data. “Data management” then enacts those policies and procedures to compile and use data for decision-making.
“Data management” are the elements for ingesting, storing, organising and maintaining the data created and collected by an organisation.
“Data quality management” is one of the elements of data management (and therefore data governance). Data quality is a measure of the condition of data based on factors such as
- accuracy,
- completeness,
- consistency,
- reliability, and
- whether it's up to date.
Webinar Watch on-demand: An Introduction to Spatial Data Governance and Management
Spatial data quality management and data governance
When it comes to managing the quality of spatial data, there are specific challenges to consider. When the data is collected (or retrieved), it needs to be transformed into an appropriate, uniform format, which can prove more difficult with spatial data because of the wide diversity of data sources and formats. Moreover, spatial data requires constant updating, because it can get out of date quicky due to its transient nature. And the sheer volume of the spatial data that is continually generated means it usually requires specific tools to manage it.
Moreover, there are several common errors within large spatial datasets that can exacerbate data quality concerns.
- positional errors (when points or objects appear at different locations than their actual location),
- attribute errors (when incorrect attributes are assigned to certain objects), and
- topological errors (when relationships between objects are inaccurate).
There are also commonly occurring data entry errors that need to be addressed, such as data transmission errors, data storage errors and data processing errors.
Read more about geospatial data and why it’s different from regular data.
Data challenges across the UK Public Sector
In 2019 the UK National Audit Office (NAO) published a report – “Challenges in using data across government”, which highlighted the challenges for government in making long-term cross-government investments. The report mentioned three key findings:
- Data is not always seen as a priority: The quality and sharing of data is a clear example of a neglected and poorly planned activity. If government is serious about data being one of its most important assets, it is long overdue a balance sheet review.
- The quality of data is not well understood. Government has pursued the benefits of better use of data, but new initiatives often expose the poor quality of the data itself. Good data is not a ‘free good’ and government needs a structured approach to investing in improving and using data.
- There is a culture of tolerating and working around poor-quality data. Evidence-based decision-making is a necessary condition for achieving value for money in public spending. And government needs to develop the capability, leadership and culture to support sustained improvement in the quality of information available.
Quality spatial data needs data governance
It’s quite clear that without a data governance “first” led approach, followed by data management, then:
- Spatial data quality management will not be enterprise-wide;
- Spatial data quality management efforts will remain localised;
- There will be a lack of common data management standards;
- There won't be clear data management roles and responsibilities;
- Spatial data quality management will be mostly reactive.
Where spatial data will be used to make critical decisions and projections, it is essential that organisations adopt a “data governance first”-led approach to ensure the spatial data is trusted, well-documented, easy to find and share, and that it continues to remain that way.
Free webinar: Achieve better data governance with 1Integrate
Join us for this practical discussion of how to achieve better data governance by improving your data management with 1Integrate.
Register now